Businesses flock to the cloud for its unmatched scalability, flexibility, and cost reductions. However, optimizing cloud costs becomes challenging as environments become complex. Human monitoring struggles to keep up with data influx, resulting in unexpected cost escalations that impact the bottom line and force engineers to add difficult tasks such as forecasting usage and adjusting allocations to their already hefty to-do list.
Thankfully, there’s no need to manage it all on our own. ML steps in to analyze vast amounts of data rapidly, making intelligent decisions for a variety of key use cases. In the context of cloud cost optimization, ML plays a vital role in:
- Analyzing usage patterns and uncovering underutilized resources. This allows organizations to rectify inefficiencies and reduce costs.
- Recommending cost-effective instance types and pricing models. ML leverages historical data to help organizations choose optimal options for greater efficiency.
- Pinpointing specific cost-driving aspects of the cloud. This enables organizations to focus optimization efforts on high-cost areas for maximum savings.
- Analyzing usage patterns to accurately predict future resource requirements.
Despite the clear advantage of using ML for cloud cost optimization, many organizations have yet to adopt this technology, or even cloud cost optimization solutions in general. Battery Ventures’ State of Cloud Spending Report shows that while 95{29fe85292aceb8cf4c6c5bf484e3bcf0e26120073821381a5855b08e43d3ac09} of CXOs consider cloud cost optimization a priority, only 55{29fe85292aceb8cf4c6c5bf484e3bcf0e26120073821381a5855b08e43d3ac09} use ML-based third-party solutions. Despite that, 31{29fe85292aceb8cf4c6c5bf484e3bcf0e26120073821381a5855b08e43d3ac09} of CXOs rank ML optimization as a #1 feature priority when it comes to selecting third party cloud cost optimization solutions.
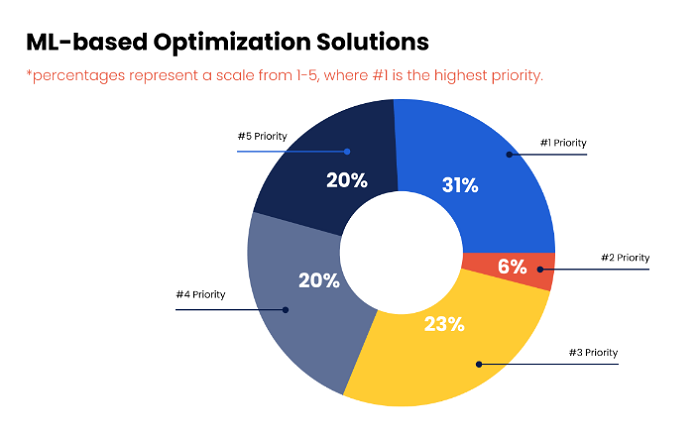
Benefits of Using ML for Cloud Cost Optimization
Although many companies have yet to fully embrace ML-based solutions, studies indicate that 86{29fe85292aceb8cf4c6c5bf484e3bcf0e26120073821381a5855b08e43d3ac09} plan to adopt these technologies within the next five years.
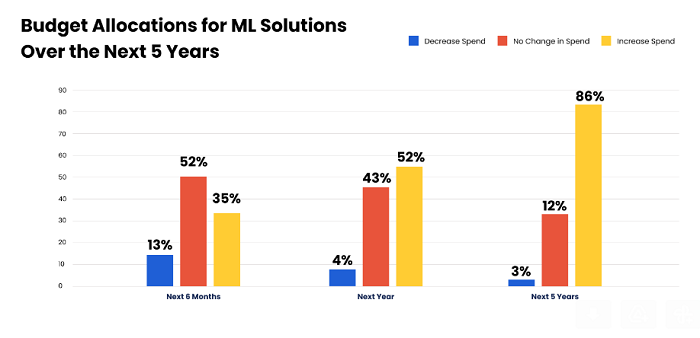
This is because incorporating ML into cloud cost optimization strategies provides an array of capabilities to improve their cloud infrastructure’s ROI, without adding pressure to their engineers.
The benefits include:
Improved Accuracy
ML algorithms analyze vast volumes of data, detecting patterns and trends that might elude human observers. This leads to more accurate cost optimization recommendations, resulting in significant cost savings.
Real-time Insights
ML algorithms can analyze data in real-time, adapting to changing usage patterns and providing immediate recommendations. Organizations can respond promptly to fluctuating demand and optimize their cloud infrastructure in real-time.
Scalability
Cloud environments can be complex and dynamic, with numerous resources and constantly changing usage patterns. ML algorithms excel at processing large-scale data, enabling cost optimization for even the most intricate cloud setups.
Automation
ML algorithms automate the identification of cost-saving opportunities and the continuous optimization of cloud infrastructure, reducing the need for manual intervention. This saves time and resources, allowing organizations to focus on strategic initiatives.
Continuous improvement
As ML algorithms analyze more data over time, they continually learn and adapt, enhancing their cost optimization recommendations and maximizing savings for organizations.
There are a large variety of tools on the market that provide ML solutions for cloud cost optimization, but they are primarily focused on visibility and recommendations. The majority of them fall under the category of “real-time insights,” pinpointing areas of excess spending and providing recommendations of how organizations can cut costs. While this is a step in the right direction, a human still needs to implement the recommendations.
Actions Speak Louder than Words
To unlock the deeper capability of ML for cloud cost optimization, automation capabilities need to be at the forefront of any ML offering. Automation can go beyond recommendations and insights, taking real-time action in order to instantly reduce costs without any effort or input from engineers.
Mere recommendations are simply not enough for today’s fast paced business environment. For this reason, organizations should use cloud cost optimization solutions which use ML algorithms to perform real-time actions that actively reduce costs on both compute and storage fronts.
This provides several key benefits:
- Maximizing Savings: ML algorithms are at work 24/7 so they can instantly respond to unexpected changes, saving significantly more than non-ML based solutions.
- Minimizing Manual Work: ML-driven automation alleviates the burden of manual cloud cost optimization tasks. Forecasting usage, adjusting allocations, and manually expanding or shrinking disks are all automated, freeing up valuable time for cloud operations teams.
- Eliminating Human Error: Costs are optimized without the risk of human error. The ML algorithms make data-driven decisions based on real-time insights, ensuring precise and accurate cost optimizations.
Final Thoughts
In the world of cloud computing, the promise of scalability and flexibility often falls short due to the static nature of provisions, in contrast to usage which is always dynamic. This mismatch has created a need for ML-driven solutions that can bridge the gap; cut cloud costs, reduce waste, and optimize resource allocation.
ML technology enables infrastructure to become more dynamic, capable of autonomously adjusting to demand. By leveraging ML to drive real-time actions, customers are able to access cloud savings that are otherwise impossible to reach and obtain.
About the Author

Maxim Melamedov is the CEO and co-founder of Zesty, an automated cloud optimization platform. With over 13 years of experience in the tech industry, including a prior career in homeland security, Melamedov thrives on solving complex problems and disrupting previously established norms. He finds endless inspiration at the intersection of data, innovation, and efficiency. Melamedov earned his bachelor of arts in business management from the University of Derby and is a veteran of the Israeli Air Force.
Sign up for the free insideBIGDATA newsletter.
Join us on Twitter: https://twitter.com/InsideBigData1
Join us on LinkedIn: https://www.linkedin.com/company/insidebigdata/
Join us on Facebook: https://www.facebook.com/insideBIGDATANOW